NIT Rourkela Develops AI-Driven Vehicle Detection System for Enhanced Traffic Management
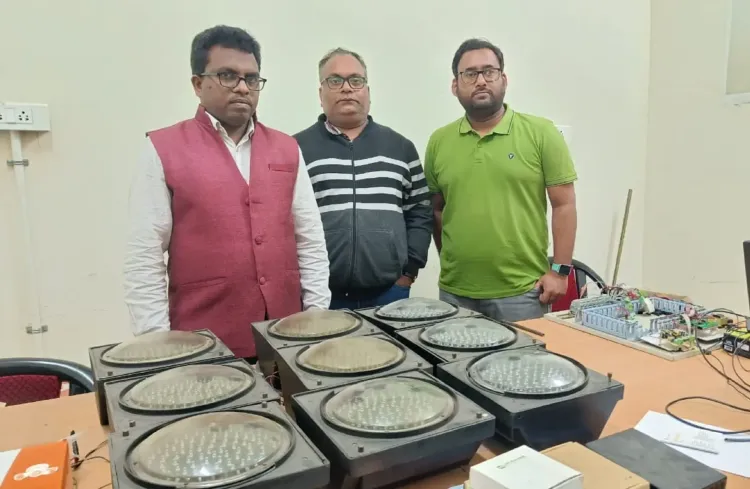
Synopsis
Key Takeaways
- AI-based vehicle detection enhances traffic management.
- Model tested with the Heterogeneous Traffic Labeled Dataset.
- Real-time performance evaluated on Nvidia Jetson TX2.
- Utilizes computer vision for vehicle identification.
- Future plans include a commercial traffic control system.
New Delhi, Jan 24 (NationPress) An Artificial Intelligence (AI)-powered multi-class vehicle detection (MCVD) model aims to enhance traffic management in developing nations like India, according to researchers from the National Institute of Technology Rourkela (NIT Rourkela) on Friday.
Published in the esteemed journal IEEE Transactions on Intelligent Transportation Systems, the MCVD model showcases an accuracy boost over existing techniques.
The team evaluated the model using the Heterogeneous Traffic Labeled Dataset (HTLD), which comprises data from multiple cities across India and is publicly accessible.
Moreover, the model's performance was tested in real-time on the Nvidia Jetson TX2, an edge computing device, maintaining strong speed and accuracy even in adverse weather conditions and with low-resolution imagery.
“By addressing the challenges of older models and the specifics of mixed traffic, the MCVD model provides a scalable solution for real-time vehicle detection in developing regions,” stated Prof. Santos Kumar Das, Associate Professor, Dept. of Electronics and Communication Engineering, NIT Rourkela.
“Its implementation could lead to enhanced traffic systems, decreased congestion, and improved road safety,” Prof. Das further commented.
The team utilized an intelligent vehicle detection (IVD) system, which employs computer vision technology to identify vehicles in images and videos. This system gathers real-time traffic data to optimize flow, mitigate congestion, and assist in future road planning.
Prof. Das and his team designed the new MCVD model, using a Video Deinterlacing network (VDnet) to effectively extract crucial features from traffic images, accommodating for varying vehicle sizes and shapes.
Additionally, they developed a specialized tool named Light Fusion Bi-Directional Feature Pyramid Network (LFBFPN) to enhance the detail extraction process.
The research group is also working on a traffic control system based on this concept and intends to commercialize it via a start-up.